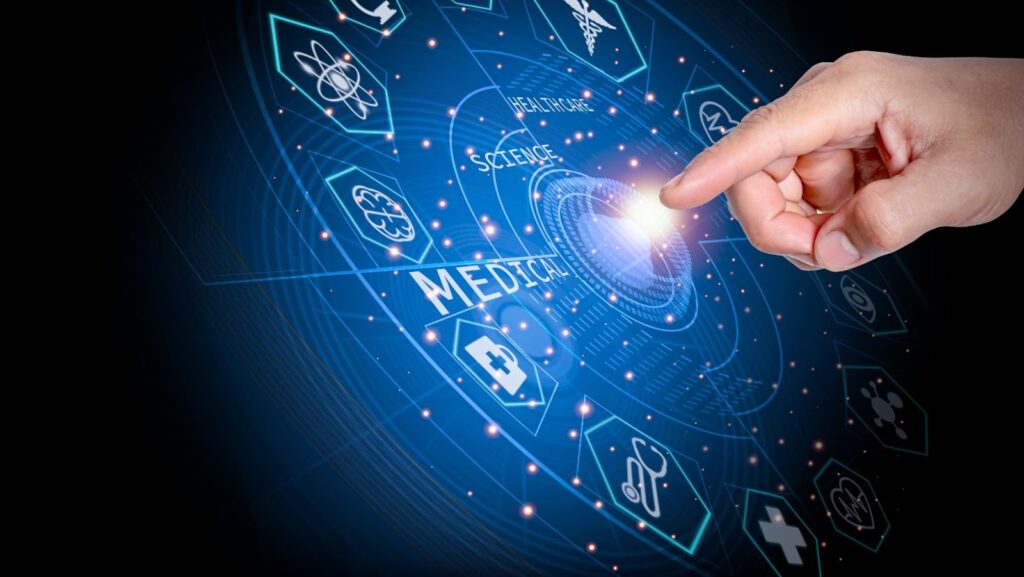
Parallel learning is an innovative approach to digital health where traditional healthcare delivery models and processes are replaced with digital mechanisms. It is an important piece in the evolution of digital health, as it enables healthcare professionals to provide better access to services and treatment options.
In this article, we will explore how parallel learning is changing digital health and how it is impacting healthcare delivery.
Definition of Parallel Learning
Parallel Learning is a form of artificial intelligence that enables medical machine algorithms to learn from the data they generate, rather than solely relying on pre-programmed rules. This type of learning is known as “self-contained” within the context of AI, and it requires minimal human input for it to learn. As a result, parallel Learning can help streamline and improve the accuracy of patient diagnosis and treatment decisions compared to traditional approaches by allowing machines to identify patterns in data with minimal intervention from a human instructor.
To maximize its potential, Parallel Learning must be applied by an underlying set of principles that inform how the algorithm learns from given data. This includes understanding underlying informational systems and constructing frameworks around them to determine relevant information and how it should be used for maximum efficiency. Additionally, there must be enough high-quality instructional feedback available for training purposes so that progress can be tracked accurately over time as well as adaptation made when necessary. By layering these principles with existing computing frameworks like supervised learning or unsupervised clustering algorithms, robust decision support systems are developed which further support informed clinical decisions while simultaneously reducing workloads and increasing efficiencies across medical teams.
Digital Health Platform Parallel Learning Serve Students with Learning and Thinking Differences
Parallel learning transfers knowledge through multiple pathways or channels to achieve a particular goal. It enables learners to acquire new skills in different ways that require less effort and are more effective. Parallel learning has become increasingly popular in the digital health world, allowing individuals and healthcare professionals to absorb information faster and more effectively.
Parallel learning has many benefits, including increased engagement, improved understanding of complex concepts, and measurement of learning objectives. In addition, learners can develop insight into their health status with greater confidence by engaging in parallel teaching – utilizing multimedia platforms such as virtual reality (VR) simulation, online discussion forums, and self-paced online instruction.
Instead of traditional classroom instruction which may not allow for adequate time for the experiment or application process; learners have uninterrupted access to explore individualized educational materials in multiple formats using this approach. This eliminates the need for physical presence in a lecture hall reducing cost associated with travel or registration fees. Moreover, real-time feedback from peers and mentors on questions encouraged during these sessions increases participant motivation levels, which further encourages interactive collaboration between students and teachers, enabling them to increase their uptake on healthcare-related information with ease for better health outcomes.
The Impact of Parallel Learning on Digital Health
Parallel learning is a new and growing trend in digital health that is helping healthcare professionals to be more efficient and effective in their tasks. It is a process of simultaneously teaching multiple learning techniques and strategies to help medical staff better learn about new technology and treatments. By leveraging the power of parallel learning, medical professionals can quickly obtain the same knowledge and understanding.
Let’s look at how parallel learning is impacting the healthcare sector.
Improved Efficiency and Access to Care
Parallel learning, or blended learning, has created opportunities for healthcare institutions to use a combination of online activities with real-time interactions between clinicians and patients to deliver better care more efficiently. Blended learning has changed how medical education is delivered, allowing medical students to learn and practice clinically relevant skills remotely.
By adopting parallel learning methods, training and patient care can be simultaneously conducted in the classroom and virtual environment in real time.
Doctors can conduct remote patient monitoring more effectively using technologies like video conferencing tools and secure messaging applications. For example, a patient’s vital signs can be monitored remotely and the patient’s health data can be transmitted securely. At the same time, the doctor can view it all in the same interface, allowing timely diagnosis of health issues and rapid access to care. This improves efficiency of treatment delivery, reduces time spent waiting for appointments, and reduces waiting times for critical tests results. In addition, these technologies provide convenience for patients who are not able to make it into an office due to geographical constraints or other physical limitations.
Increased Engagement and Patient Outcomes
Parallel learning transforms digital health by creating a more engaging and impactful patient experience. By increasing patient engagement, parallel learning can lead to improved outcomes.
Parallel learning uses a combination of technology, such as computers and mobile devices, to create an environment where healthcare providers and patients can interact in real-time using online tools. This type of shared knowledge helps to foster collaboration that can lead to meaningful conversations about the patient’s condition and progress.
By providing up-to-date information and resources, parallel learning enables healthcare providers to better identify the most appropriate interventions for the individual’s needs. In addition, through interactive features such as chatbots or virtual assistants, patients can engage with their care provider effortlessly from anywhere. This type of personalization helps build trust between the patient and care provider, often leading to improved patient compliance and outcomes.
Overall, parallel learning is revolutionizing digital health by allowing healthcare providers and their patients to collaborate more effectively through increased engagement, leading to better health outcomes.
Reduced Costs and Improved Quality
Parallel learning is revolutionizing the field of digital health. By combining the knowledge and expertise from traditional medical care to predictive analytics, machine learning and mobile applications, parallel learning can reduce healthcare costs and improve healthcare quality.
Adopting parallel learning methods in digital health also helps provide personalized care with greater accuracy. By combining data points related to lifestyle, behavior and physical condition, providers can more accurately share information. This allows more time to be focused on patient care rather than collecting data or verifying records—ultimately reducing medical errors. In addition, personalized data can be combined with knowledge accumulated through observation-based clinical studies to provide even better insight into treatment options for each patient.
As parallel learning continues to grow in medicine, AI-powered systems are being used to interpret patient information quickly and accurately so that providers and patients can make informed decisions about their treatment options. In addition, clinical decision support systems based on real-time data extrapolated from existing medical records and environmental conditions help predict outcomes more accurately than previous methods.
The adoption of technologies such as telemedicine or remote patient monitoring helps keep costs under control. In addition, it improves quality of care, improving patient outcomes worldwide by leveraging actionable insights that wouldn’t normally be available from traditional care pathways alone.
Challenges of Implementing Parallel Learning
Parallel Learning promises to revolutionize digital health by providing more efficient, personalized and cost-effective care. But, it’s not without its challenges.
This section will identify some challenges in implementing Parallel Learning and outline strategies for overcoming them.
Lack of Standardization
One of the main challenges associated with implementing parallel learning methods is the lack of a standardized architecture or process to build and store communally shared concepts. Depending on an organization’s goals, desired outcomes and user requirements may change over time, and effective coordination and communication among all stakeholders can become difficult. Additionally, it may be difficult to know who is responsible for creating which datasets and how incoming information should be stored and retrieved for use by other stakeholders.
Parallel learning is hindered by the scarcity of uniform platforms designed to share information among digital health departments. In addition, as more and more organizations create their digital health systems, the challenge of creating interoperable frameworks suitable for digital exchange becomes increasingly daunting. This can lead to inefficiencies when data is exchanged from one system to another, resulting in a lack of data integrity due to incompatibility issues. Encouraging organizations to adopt common interoperability standards can help streamline communications between disparate systems; however, widespread adoption remains a long-term goal due to complexities involved in consolidating data within multiple systems into one consistent format.
Data Security and Privacy Concerns
The nature of healthcare and data security and privacy concerns make implementing parallel learning a challenge. The high sensitivity of health-related data requires robust security measures that ensure data is encrypted, secure, and only accessible to the right people.
Furthermore, laws like HIPAA and General Data Protection Regulation (GDPR) must strictly maintain patient privacy and health information confidentiality. This means organizations must take appropriate measures to secure all aspects of the digital space where such information is gathered. These include ensuring regulated authentication needs for accesses and secure communication protocols between applications.
As parallel learning systems become increasingly sophisticated in uncovering patterns from numerous sources of patient information, further precautionary steps are needed to protect sensitive relevant data from malicious actors. For this reason, parallel learning has prompted public sector entities and organizations providing services to develop a stringent process for consuming, accessing and auditing the stored health-related data.
Limited Resources
Parallel learning initiatives face the challenge of limited resources to fully implement and maximize its impact. Organizations leading in this field, such as the International Association of Medical Research Institutes (IAMRI) are working to combat this issue by providing resources and standardizing practices across a wide range of data sets.
Another challenge is cost-effectiveness as new technologies are expensive and often require additional support infrastructure. Additionally, though many solutions can be scaled up, they may lack sufficient capacity to deal with concurrent requests or manipulate large datasets in real time.
Moreover, there can be a lack of awareness about the potential benefits of Parallel learning solutions for medical organizations. In addition, many medical professionals may be hesitant to adopt new technologies due to worries regarding data security and privacy issues—as digital health advancements come with an increased risk for cyberattacks. Lastly, organizations must also consider cultural aspects when implementing any new AI solution, ensuring that the technology fits in with their existing values and policies while creating an environment beneficial to employees at all levels.
Ultimately, all stakeholders must strive towards successfully deploying digital health solutions, simultaneously addressing system and personnel-related challenges.
Examples of Parallel Learning in Digital Health
Parallel learning is a formal learning and assessment process that takes place concurrently with other activities and can happen simultaneously with treatment, work, leisure and recreation. It is becoming increasingly more popular in the digital health space, allowing for the simultaneous collection of patient data and helping improve care outcomes.
Here, we will discuss examples of how parallel learning transforms digital health.
Telemedicine
Telemedicine is an increasingly popular form of remote healthcare delivery that uses digital technologies to provide clinical care at a distance. Through telemedicine, clinicians can diagnose, treat and monitor patients more conveniently and effectively. In addition, by utilizing parallel learning technologies such as telemedicine, educational institutions can provide quality healthcare education accessible and enhanced by digital tools.
The use of telemedicine has proven to be beneficial for many fields in the digital health sector. It has been used to improve the quality and accuracy of diagnoses, treatments and follow-up care while exposing healthcare professionals to new expertise and skillsets they can apply daily. Moreover, by allowing patient visits without being physically present – through video calls or consultations over the web – clinicians can reduce hospital visits and increase their time efficiency while improving the overall quality of care provided.
To make the most out of parallel learning through telemedicine various principles need to be adhered to: Firstly, valuable data needs to be gathered via patient observation – this data should include case studies that illustrate best practices while also identifying areas of improvement or further research that might enhance existing strategies or treatments; Secondly, a definite goal needs to be established with clear objectives as well as measurable targets for performance; Thirdly and finally, adaptive learning measures should be utilized for continuous development within an ever-evolving digital health sector.
Wearable Devices
Wearable devices and sensors are becoming increasingly popular as a form of parallel learning, as they can easily provide vast amounts of data about someone’s health and lifestyle. By monitoring physical activity, eating behaviors and rest patterns, this type of technology provides feedback based on real-time data from the user that can be used to adjust lifestyle factors to improve an individual’s overall health.
The potential for wearable tech lies in its ability to detect early signs of health deterioration or changes in behavior before it becomes a major issue. For example, suppose someone has problems sleeping or has developed high blood pressure due to stress. In that case, wearable device apps could detect subtle changes over time and make personalized suggestions or recommendations that might resolve the issue.
Wearables also play a role in long-term non-communicable diseases such as diabetes through advanced sensors that measure glucose levels. Wearable devices can collect regular readings of blood sugar levels by continuously tracking biometric data, allowing health practitioners and individuals alike to monitor trends over time and take necessary action when necessary. Additionally, researchers have investigated how wearables can be used for mental health applications such as depression or PTSD via audio training and conversation analysis.
Overall, wearables offer an insight into our lifestyles and mental state — which helps us make smarter decisions about our health and wellbeing. As these devices become more widely adopted due to their affordability and convenience, the potential for “parallel learning” is undeniable — both for consumers who want to stay on top of their physical conditioning and researchers who want insights into population health trends.
Artificial Intelligence
Artificial intelligence is quickly becoming a staple technology in the digital health arena and it is also transforming into one of the most important tools for parallel learning. AI uses sophisticated algorithms and machine learning to recognize patterns and draw insights from data. By leveraging this technology, healthcare providers have the potential to generate personalized patient care plans using more accurate prognostic conditions, as well as facilitate automated diagnosis, improving diagnosis accuracy and precision in clinical decision making.
Furthermore, it can also be used to enable remote sensing and monitoring of patient health from afar. AI-driven technologies such as wearable devices, smartphone applications and remote sensors can track biometrics vital to health such as heart rate, oxygen saturation level and temperature, alert healthcare professionals to any potential risks or danger signs before a medical emergency. This can help health care providers quickly address urgent issues or diseases through timely interventions.
Moreover, AI-enabled predictive analytics techniques could help healthcare providers identify early signs of disease based on population data and recommend appropriate preventative measures or treatments for individualized care-plan decisions on patients enrolled in population/precision health programs that utilize real-time patient data collected on an ongoing basis. As a result of this process, threats posed by acute diseases can be minimized drawing upon the power of AI-enabled predictive analytics customized for each person’s specific needs.
Conclusion
Parallel learning is having a major impact on digital health. Not only is it creating a seamless process for patients to access their information and receive care from healthcare providers, but it’s also making it possible for technology to better connect patients with providers. This could have a far-reaching impact on healthcare, making it more efficient and effective.
In conclusion, parallel learning transforms digital health and creates a more connected healthcare system.
Summary of Benefits and Challenges
Parallel learning approaches have quickly become integral to digital health strategies and envisioning use cases. With improved accuracy, they can detect patterns of natural language and unearth insights faster than ever before achieved. This process makes it easier for healthcare providers to make evidence-based decisions and remain informed regarding emerging trends in medical science. On top of that, machine learning technology enables personalized healthcare experiences that cater to individual health needs by leveraging patient data for treatment plans.
However, artificial intelligence (AI) and its related applications present challenges like every other technology. These range from data privacy & security issues caused by algorithms finding unauthorized accesses to patient information, to bias errors caused due to inherent limitations in the system’s ability to understand dynamic contexts. Thus, conscientious steps must be taken from healthcare administrators and regulators while utilizing these tools and ensuring they adhere to established laws & regulations governing the use of AI in healthcare settings.
Future of Parallel Learning in Digital Health
Parallel learning is increasingly being used to bridge the gap between data and recommendations for healthcare. As technologies like machine learning evolve, parallel learning will play an even more significant role in improving digital health.
Its ability to personalize medicine is a key factor that will drive parallel learning forward in the medical field. By combining different health data sources, such as previous treatments and genomic information, parallel learning can provide personalized care plans and better outcomes. Additionally, its approach to analyzing large datasets will enable medical researchers to discover new insights on treatments, diseases and potential cures more efficiently.
As more healthcare providers recognize the benefits of using parallel learning in their practices, they can streamline operations and create better patient experiences. In addition, by incorporating an AI-assisted decision making process into their systems, patients receive real-time solutions tailored to meet their needs sooner rather than later. This could ultimately lead to reduced costs associated with managing chronic conditions and providing effective care for long-term illnesses.
By harnessing and leveraging the power of data science and technology advances such as machine learning algorithms, Parallel Learning can transform healthcare decisions without disrupting existing workflows or compromising patient safety standards. As AI tools become faster, smarter & pervasive – they will continue to define significant changes in how clinicians treat patients while providing a quantifiable competitive edge over other healthcare providers in the market through improved efficiency & high quality health outcomes.
tags = Digital Health Platform, Parallel Learning, $20M, Expand and Serve Students with Learning and Thinking Differences, new yorkbased parallel tiger globalcoldeweytechcrunch, digital health platform, Tiger Global, licensed psychologists, learning specialists, behavioral health therapists and speech therapists