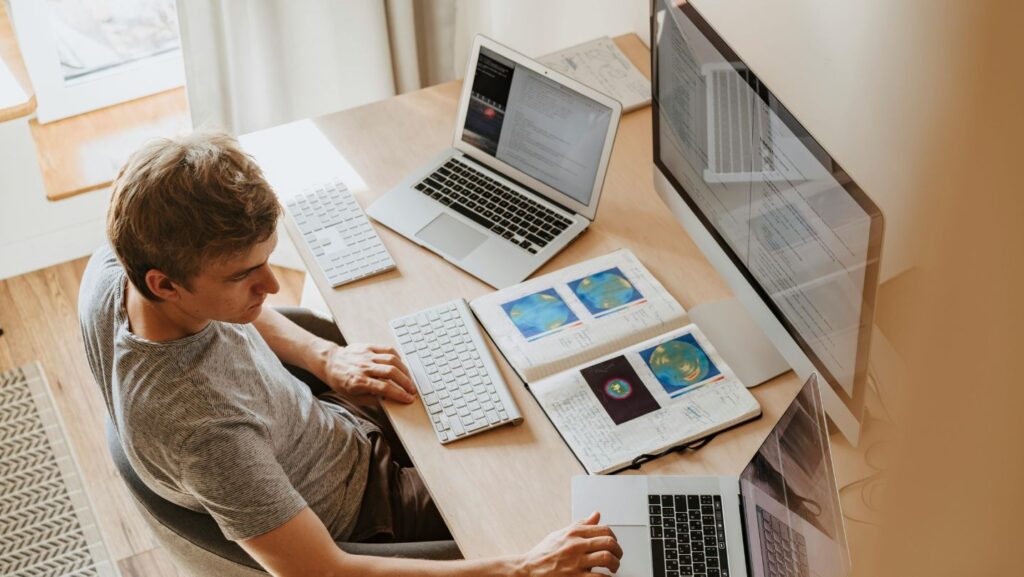
The technology behind decision support systems (DSS) has evolved significantly, providing organizations with powerful tools to analyze data, forecast trends, and make strategic choices. This article explores the core technologies driving decision support in modern business, including data analytics, artificial intelligence (AI), machine learning (ML), and advanced visualization tools.
Data Analytics: The Foundation of Decision Support
Data analytics is the backbone of decision support technology. It involves collecting, processing, and analyzing vast amounts of data to uncover insights that guide business decisions. Modern data analytics platforms enable organizations to handle both structured and unstructured data from various sources, such as customer interactions, sales transactions, and market trends.
Big Data technologies, like Hadoop and Spark, play a significant role in this process. They allow businesses to store and process enormous datasets efficiently. By leveraging these technologies, companies can identify patterns, correlations, and trends that were previously difficult to detect. This insight supports decision-making across various business functions, from marketing strategies to supply chain management.
Decision-Making Software: Streamlining Processes
In addition to data analytics, decision making software plays a crucial role in modern decision support systems. These platforms integrate various technologies to streamline the decision making process, offering a comprehensive suite of tools for data analysis, scenario planning, and strategic forecasting.
Decision making software often includes features like simulation models, risk assessment tools, and decision trees, which help businesses evaluate different strategies and outcomes.
By incorporating these tools, organizations can assess potential scenarios and their impacts before making critical decisions. This capability is especially valuable in complex environments where multiple variables and uncertainties must be managed.
Artificial Intelligence: Enhancing Decision-Making
Artificial Intelligence (AI) has become a game-changer in decision support systems. AI algorithms can analyze large datasets at high speeds, providing actionable insights that drive strategic decisions. Machine learning, a subset of AI, further enhances these capabilities by enabling systems to learn from historical data and improve over time.
Predictive Analytics is a key application of AI in decision support. By using historical data to forecast future trends, businesses can anticipate market changes, customer behavior, and potential risks. For example, retail companies use predictive analytics to optimize inventory levels, ensuring they meet customer demand without overstocking.
AI-powered tools also facilitate Natural Language Processing (NLP), allowing decision-makers to interact with data using natural language queries. This technology simplifies data analysis by enabling users to ask questions and receive insights without needing deep technical expertise.
Machine Learning: Automating and Optimizing Decisions
Machine learning (ML) algorithms are designed to automatically identify patterns and make decisions based on data. In decision support systems, ML models can analyze data more deeply and accurately than traditional methods. These models can be supervised, unsupervised, or reinforcement-based, each serving different purposes in decision-making processes.
Supervised Learning involves training models on labeled datasets to predict outcomes or classify data. For instance, financial institutions use supervised learning to detect fraudulent transactions by identifying patterns indicative of fraud.
Unsupervised Learning, on the other hand, is used to find hidden patterns or groupings in data without predefined labels. This approach is useful for customer segmentation, where businesses group customers based on purchasing behavior and preferences.
Reinforcement Learning focuses on training models to make decisions through trial and error. It is particularly valuable in dynamic environments where continuous adaptation is required, such as in automated trading systems or recommendation engines.
Advanced Visualization Tools: Making Data Accessible
Data visualization tools are essential for making complex data accessible and understandable. Modern decision support systems use advanced visualization techniques to present data in intuitive and interactive formats. These tools help decision-makers grasp insights quickly and make informed choices.
Dashboards are a popular visualization tool that aggregates key performance indicators (KPIs) and metrics in a single view. They provide real-time updates and allow users to drill down into specific data points for deeper analysis.
Geospatial Analytics is another advanced visualization method that maps data to geographic locations. This approach is particularly useful for businesses with a geographical component, such as retail chains or logistics companies, as it helps optimize site selection, route planning, and market analysis.
Interactive Reports and data storytelling techniques further enhance decision support by presenting data in a narrative format. These tools enable users to explore data dynamically and understand the context behind the numbers, facilitating more informed decision making.
Conclusion
The technology behind decision support systems has transformed how modern businesses approach decision making. By leveraging data analytics, decision making software, artificial intelligence, machine learning, and advanced visualization tools, organizations can gain valuable insights, anticipate future trends, and make strategic choices with greater confidence. As technology continues to evolve, the capabilities of decision support systems will undoubtedly expand, offering even more sophisticated tools for navigating the complexities of the business landscape.